AI data analysis involves using artificial intelligence to extract insights and make informed decisions from large datasets efficiently. Businesses can automate data analysis processes by leveraging AI algorithms and uncovering valuable trends and patterns.
From predictive analytics to natural language processing, AI empowers organizations to derive actionable insights and drive strategic decision-making based on data-driven intelligence. Through advanced technologies like machine learning and deep learning, AI can revolutionize how businesses interpret and utilize data for improved outcomes and competitive advantage in today’s data-driven landscape.
Whether it’s optimizing sales strategies or enhancing operational efficiency, AI-driven data analysis is a powerful tool for unlocking the full potential of data assets and driving business growth.
Table of Contents
AI Analysis Of Data
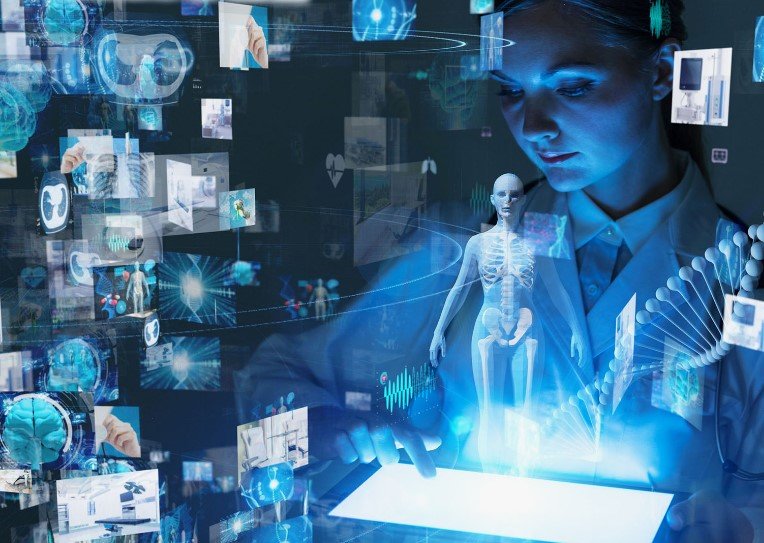
The Evolution Of AI in Data Analysis
Over the years, the field of data analysis has witnessed a remarkable transformation with the integration of Artificial Intelligence (AI). This evolution has revolutionized how organizations leverage data to gain insights and make informed decisions.
From Traditional Methods To AI Integration
The shift from conventional data analysis methods to AI-driven techniques has significantly enhanced the efficiency and accuracy of analyzing vast and complex datasets. With AI, tasks that once required manual intervention and extensive time can now be automated, allowing for faster and more precise data processing.
Benefits Of AI in Data Analysis
- Enhanced Accuracy: AI algorithms can analyze data with a higher degree of precision, reducing the margin of error in decision-making.
- Improved Efficiency: AI data analysis frees up valuable human resources by automating repetitive tasks and enabling professionals to focus on strategic analysis.
- Advanced Insights: AI algorithms can uncover hidden patterns and trends in data that might go unnoticed with traditional methods, leading to more insightful conclusions.
Traditional Methods | AI Integration |
---|---|
Manual data processing | Automated data analysis |
Time-consuming analysis | Real-time data insights |
Limited scalability | Scalable data processing |
AI in data analysis has opened up new possibilities for organizations to extract valuable insights from their data, leading to more informed decision-making and competitive advantages in today’s data-driven world.
Applications Of AI in Data Analysis
AI has revolutionized the way data is analyzed, providing innovative solutions that drive efficiency and accuracy. From machine learning algorithms to natural language processing and predictive analytics, AI offers a multitude of applications in data analysis. Let’s delve into these applications in more detail.
Machine Learning Algorithms
Machine learning algorithms play a pivotal role in data analysis, enabling systems to learn from data and make predictions or decisions. By utilizing complex algorithms and statistical models, AI can identify patterns within datasets, leading to enhanced insights and informed decision-making.
Natural Language Processing
Natural Language Processing (NLP) empowers AI to understand, interpret, and generate human language, enabling it to analyze unstructured data such as text and speech. Through sentiment analysis, entity recognition, and language translation, NLP facilitates comprehensive data analysis, unlocking valuable information from textual data.
Predictive Analytics
Predictive analytics leverages AI to forecast future outcomes based on historical data, enabling businesses to anticipate trends, mitigate risks, and optimize strategies. By employing machine learning and statistical techniques, predictive analytics empowers organizations to make data-driven decisions, enhancing operational efficiency and competitiveness.
Challenges In Implementing AI for Data Analysis
Implementing AI for data analysis poses challenges such as data quality, interpretability, and bias. Ensuring accurate training data and transparent algorithms is crucial. Additionally, addressing ethical concerns and maintaining human oversight is essential for successful AI-powered data analysis.
Artificial Intelligence (AI) has revolutionized the way data analysis is performed. However, its implementation comes with various challenges that organizations need to address to leverage the full potential of AI in data analysis. Two significant challenges in implementing AI for data analysis are Data Privacy and Security Concerns and Lack of Skilled Professionals.
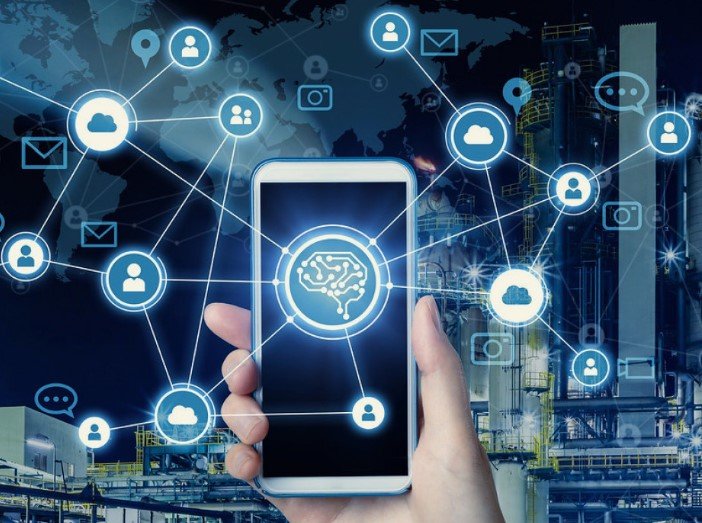
Data Privacy And Security Concerns
Data privacy and security are major concerns when it comes to implementing AI for data analysis. Organizations need to ensure that sensitive information is protected and that the AI analysis generator is compliant with data privacy regulations. With the vast amount of data being processed, AI data analysis in Excel poses a risk if not managed properly.
Lack Of Skilled Professionals
The lack of skilled professionals in the field of AI and data analysis is a significant hurdle for organizations. Automating data analysis using artificial intelligence requires professionals with expertise in AI and data science. The demand for skilled individuals often exceeds the supply, making it challenging for organizations to build and maintain a proficient AI analysis team. These challenges emphasize the need for a strategic approach to implementing AI for data analysis, ensuring that data privacy and security concerns are addressed, and investing in the development of skilled professionals in the field of AI and data analysis.
Best Practices For AI-driven Data Analysis
AI-driven data analysis best practices involve leveraging advanced AI algorithms to uncover valuable insights from complex datasets. By ensuring data quality, employing robust AI models, and interpreting results effectively, businesses can optimize decision-making and drive competitive advantages. Harnessing AI for data analysis empowers organizations to unlock hidden patterns and trends, leading to more informed strategic actions.
Best Practices for AI-Driven Data Analysis Artificial Intelligence (AI) is rapidly transforming the way businesses operate and make decisions. AI-driven data analysis has the potential to revolutionize how organizations extract insights from their data. However, to achieve optimal results, it is essential to follow best practices for AI-driven data analysis. In this section, we will explore three crucial best practices, including data quality assurance, interpretable and transparent models, and continuous learning and improvement.
Case Studies In AI Data Analysis
AI data analysis has revolutionized various industries, providing valuable insights and driving informed decision-making. Through advanced algorithms and machine learning, AI technologies are transforming the way data is analyzed and utilized. Let’s delve into some compelling case studies showcasing the impact of AI data analysis in different sectors.
Healthcare Industry
In the healthcare sector, AI data analysis has significantly improved patient care and operational efficiencies. By leveraging AI algorithms, healthcare providers can analyze large volumes of patient data to identify patterns, predict disease outbreaks, and personalize treatment plans. For instance, AI-powered diagnostic tools have demonstrated remarkable accuracy in detecting medical conditions, leading to early intervention and improved patient outcomes.
Financial Sector
AI data analysis has transformed the financial sector by enabling institutions to enhance risk management, detect fraud, and optimize investment strategies. Through predictive analytics, AI algorithms can assess market trends, identify potential risks, and recommend tailored financial products for clients. Moreover, AI-driven chatbots are streamlining customer interactions and providing personalized financial advice, enhancing customer satisfaction and retention.
Future Trends In AI Data Analysis
Explainable Ai
Explainable AI, often abbreviated as XAI, is set to play a pivotal role in the future of AI data analysis. As AI continues to evolve and become more complex, businesses and organizations must understand the reasoning behind AI-driven decisions. Explainable AI aims to provide transparency and interpretability in AI systems, allowing users to comprehend the logic and factors influencing the analysis results.
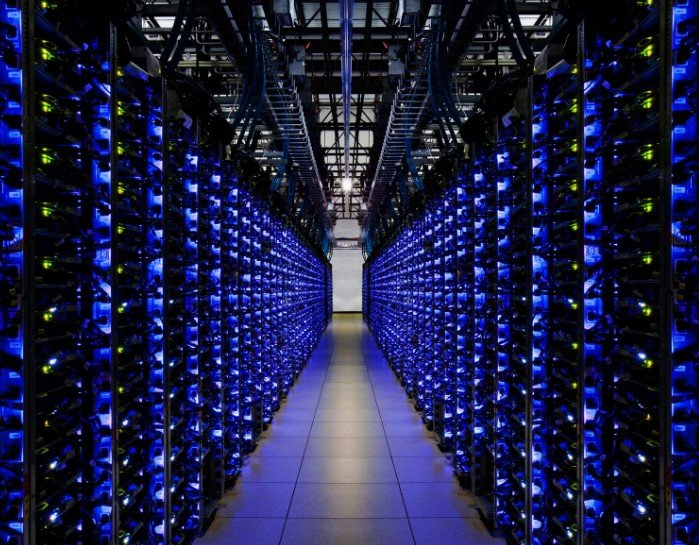
Automation Of Data Analysis Processes
The automation of data analysis processes through AI is poised to revolutionize the way businesses harness insights from their data. With AI-driven automation, tasks such as data cleaning, pattern recognition, and predictive modeling can be streamlined and accelerated, enabling organizations to make data-driven decisions more efficiently and effectively. This trend is reshaping the landscape of data analysis, empowering businesses to unlock the full potential of their datasets.
Ethical Considerations In AI Data Analysis
Exploring ethical considerations in AI data analysis is crucial for ensuring unbiased results and maintaining data privacy. Transparency and accountability are key principles in AI analysis of data to build trust and uphold ethical standards in decision-making processes. Integrating ethical guidelines into AI data analysis practices can lead to more reliable and responsible outcomes.
Ethical Considerations in AI Data Analysis As the use of AI analysis generators and AI tools for data analysis becomes more prevalent, it is important to address the ethical considerations surrounding AI data analysis. The use of automated data analysis using artificial intelligence has the potential to revolutionize the way we understand and utilize data. However, there are concerns regarding bias, fairness, accountability, and transparency that must be addressed to ensure that AI data analysis is used responsibly and ethically.
Conclusion
In the ever-evolving landscape of data analysis, AI technology continues to revolutionize the way we interpret information. With AI-driven tools like AnswerRocket and ChatGPT, complex data sets are easily translated into actionable insights. Embracing AI for data analytics opens new possibilities for automated processes and enhanced decision-making capabilities.